On May 13, Open AI launched GPT-4o, their new flagship model that can reason across audio, vision, and text in real time and the next day on May 15 the scientists leading superalignment team at OpenAI, Ilya Sutskever and Jan Leike, departed from the company. While I am impressed by the future of human-tech interactions, I can’t help but wonder what influence Open AI and enterprises alike will have over the information the modern society consumes. The global information exchanged via news, social media and even books is heavily polarized, fragmenting our knowledge of the world into small disconnected and biased information silos. Much of AI at our disposal for use is trained with different versions of these data and shown to exhibit a similar societal biases present in the data.
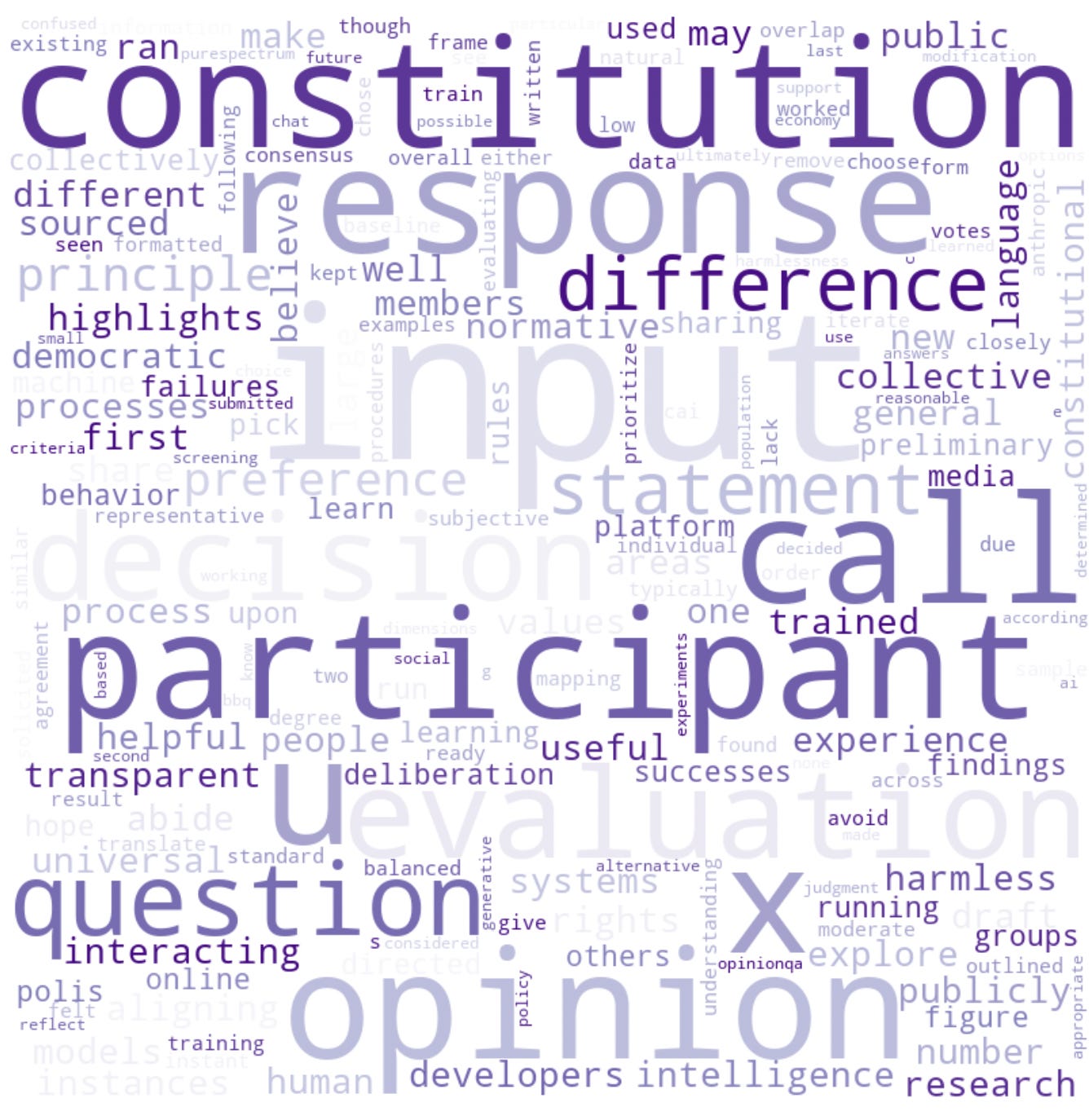
In this opinion piece, I will discuss the bias AI models encode and how it impacts society. I will also briefly talk about how firms like Open AI, Meta, Google and Anthropic are taking ethics and safety into considerations when training and deploying AI models. Drawing from the recent research and news, I will evaluate how the narrow lens of global tech might still fall short in developing AI for all. Finally, I will discuss AI regulations around the world and touch a little upon how the global south is behind in terms of making the best use of AI systems.
What is bias?
Bias, in the context of AI, means prejudices that influence AI decision-making, which leads to unfair outcomes that favors one group over another. These biases can stem from different sources: data used to train the AI, design of the algorithms and biases of the developers.
It is everywhere!
“Bias occurs in many algorithms and AI systems — from sexist and racist search results to facial recognition systems that perform worse on Black faces.
Biases are not exclusive to AI models. Every form of information we consume has been filtered through the perceptions and interpretations of an individual or a group of individuals. From the books/articles we read to videos we consume, every piece of information carries the imprint of its source. Even the education system and institutions impart knowledge, influenced by cultural, societal, and historical contexts. The weights and biases shaping artificial intelligence reflect the predispositions of the humans involved in its development along with disparity in representation present in online data sources. This disparity could stem from unequal access to the internet, varying propensities to discuss topics openly or could simply be a selection bias among others.
Regardless of the source, AI biases cast an increasingly far-reaching impact as these models are now starting to penetrate crucial areas like education, healthcare, and the legal system. Now AI is no longer just shaping our perceptions of the world around us, it now directly influences decisions that change people’s lives.
From societal representations to encoded beliefs
“The world according to Stable Diffusion is run by White male CEOs. Women are rarely doctors, lawyers or judges. Men with dark skin commit crimes, while women with dark skin flip burgers.
- Humans Are Biased. Generative AI Is Even Worse by By Leonardo Nicoletti and Dina Bass for Bloomberg Technology + Equality
Existing studies have shown that AI encodes human-like biases [1, 2, 3, 4]. A more recent study by Bloomberg on Stable Diffusion, shows that AI models no longer just mirror societal representation, they are in fact distorted and show biases worse than what exists in the society. Currently, the data used to train AI is skewed towards the viewpoints of majority groups, often overshadowing marginalized communities. This perpetuates biased narratives that disadvantage vulnerable groups, reinforcing the inequalities and biases present in the society.
While there are structured processes to measure these biases, debiasing the AI models, especially those deployed to across different communities, is hard as the notion of right and wrong is itself fluid, and evolving over time - differently across cultures and regions.
“the idea of correcting even prejudiced biases is also problematic. That is because societal understanding of prejudice is constantly evolving, along with our understanding of humanity and human rights, and also varies between cultures. It is therefore hard or impossible to specify algorithmically what is prejudiced.
- Semantics derived automatically from language corpora contain human-like biases
The nature of biases in our society and hence the data we have is complex and multifaceted. A holistic view and approach at eliminating biases from models trained on such data is hard and sometimes attempts at correcting these models, while only looking at the problem from a limited sight, can result in unintended encoding of biases from humans who create these models. The recent Gemini incident is an example of impact such tunnel vision can have as Google's overcorrection resulted in the model generating inaccurate and problematic portrayal of the history of the world.
Who is regulating and what?
It is an accepted consensus among developers, researchers and consumers of these models that there need to be guardrails in place to regulate biases in models. But, what should the regulations look like and who gets to decide those? The big names in the space, Open AI, Meta, Google, and Anthropic all have one recommendation when it comes to developing AI systems responsibly: engaging a diverse set of users throughout the development of these systems.
“Through regular collaboration with subject matter experts, policy stakeholders and people with lived experiences, we’re continuously building and testing approaches to help ensure our machine learning (ML) systems are designed and used responsibly.
- Driven by our belief that AI should benefit everyone by Meta
“We believe that many decisions about our defaults and hard bounds should be made collectively, and while practical implementation is a challenge, we aim to include as many perspectives as possible.
- How should AI systems behave, and who should decide? by Open AI
“Engage with a diverse set of users and use-case scenarios, and incorporate feedback before and throughout project development. This will build a rich variety of user perspectives into the project and increase the number of people who benefit from the technology.
- Responsible AI practices by Google
Anthropic and the Collective Intelligence Project recently conducted a public input process involving approximately 1000 Americans to formulate a constitution for an AI system. Although this is a great initiative, it falls short of representing the global user base of systems like Claude, as emphasized by Anthropic. Much like how the American constitution may not be suitable for the rest of the world, an AI constitution derived from the input of 1000 Americans is unlikely to be universally applicable. In fact, it may not even be effective for a country as diverse as the United States.
“The United States leads China, the EU, and the U.K. as the leading source of top AI models. In 2023, 61 notable AI models originated from U.S.-based institutions, far outpacing the European Union’s 21 and China’s 15.
- Artificial Intelligence Index Report 2024 by HAI Stanford
With just a few key players, mostly coming from developed regions like the US, China, and Europe, it is no surprise there is a narrow outlook in terms of how these models should behave, leaving out perspectives and considerations from other parts of the world when developing AI models.
Global governance for global systems
A survey* by Stanford researchers and Accenture found that 88% of over 1,000 global organizations believe the entities developing foundation models should be responsible for mitigating all associated risks, putting more responsibility on the model developers. However, in addition to this expectation that AI creators should be accountable in addressing biases in their AI models, 86% of respondents also recognized a need for global governance frameworks to address the broader risks involved with generative AI.
Note: the full survey* is not available yet but Artificial Intelligence Index Report 2024 by HAI Stanford reports some of the findings from the survey.
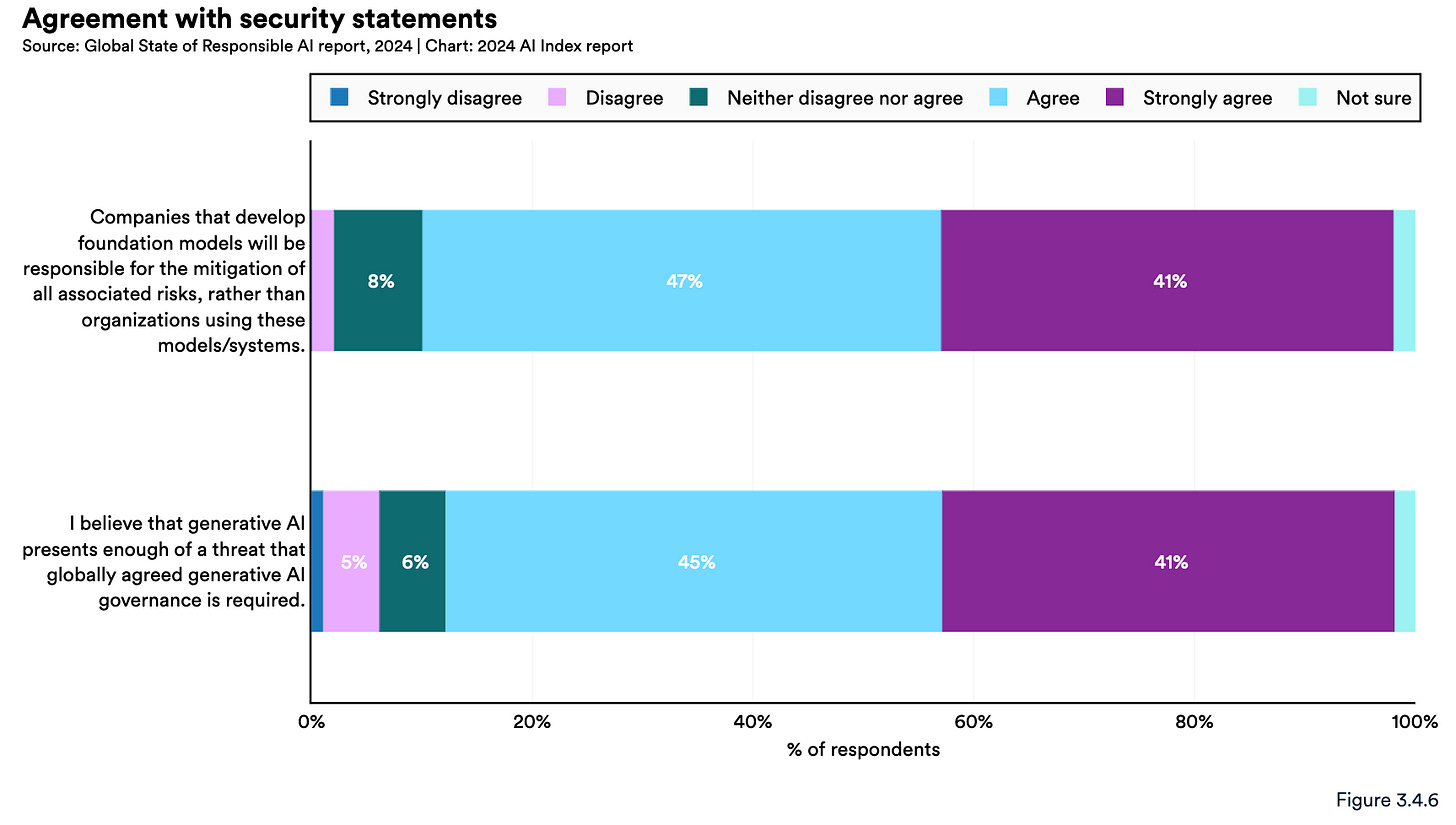
Given how the majority of the corporations leading the advancements in AI inclined towards the gated access of these models, it is natural that global consumers and organizations want greater accountability from the model developers and stricter governance frameworks to mitigate risks associated with these models. However, relying on the makers of AI too heavily to regulate the capabilities of these models would mean concentrating the power in the hands of a few monopolistic tech giants that have the resources to develop these sophisticated AI models.
“Until 2014, most significant machine learning models were released by academia. Since then, industry has taken over. In 2022, there were 32 significant industry-produced machine learning models compared to just three produced by academia.
- Artificial Intelligence Index Report 2024 by HAI Stanford
Today the majority of AI development effort is led by the industry, most of which are proprietary models. Much of what goes into development of these models, starting with data, pretraining and post-training practices are not known to the public. While all these companies have talked about their intention of building responsible AI, their prioritization does not seem to align with this intention and the newer iterations of models still display similar biases and harmful contents as their predecessors.
“Safety and security teams are being downsized or sidelined to bring AI products to market.
In the fierce race to release the next best model, ethical considerations in AI development often take a back seat. Safety and security teams are often viewed as cost centers, compliance burdens even. Hence, it is important to have multi-party stakeholders making decisions on the values and principles that should guide how AI systems should be built and how they should behave.
AI regulations around the world
“Countries and governments tend to align national priorities with their approaches to AI regulation. As a result, the flavor of ethical AI policies issued by current leaders in AI regulation - namely, the U.S., EU, and China - are very different.
- Responsible and Safe AI: A Primer for Policymakers in the Global South by Igarapé Institute
In the recent year we have seen a growth in the number of AI regulations and recommendations from nations, economic blocs, experts and non-profits organization. However, these policies and guidelines are shaped by the respective priorities and value systems of each nation or region, resulting in distinct approaches to AI regulation. This, I believe, works well, as localized AI policy can provide a more nuanced approach and regulations that comply with different culture, values, and economic goals of the country. One concern with localized AI policy is that this can lead to fragmentation in the regulatory landscape. Additionally, "developing world faces several disadvantages that make it more difficult for countries to formulate and enforce responsible AI policies."
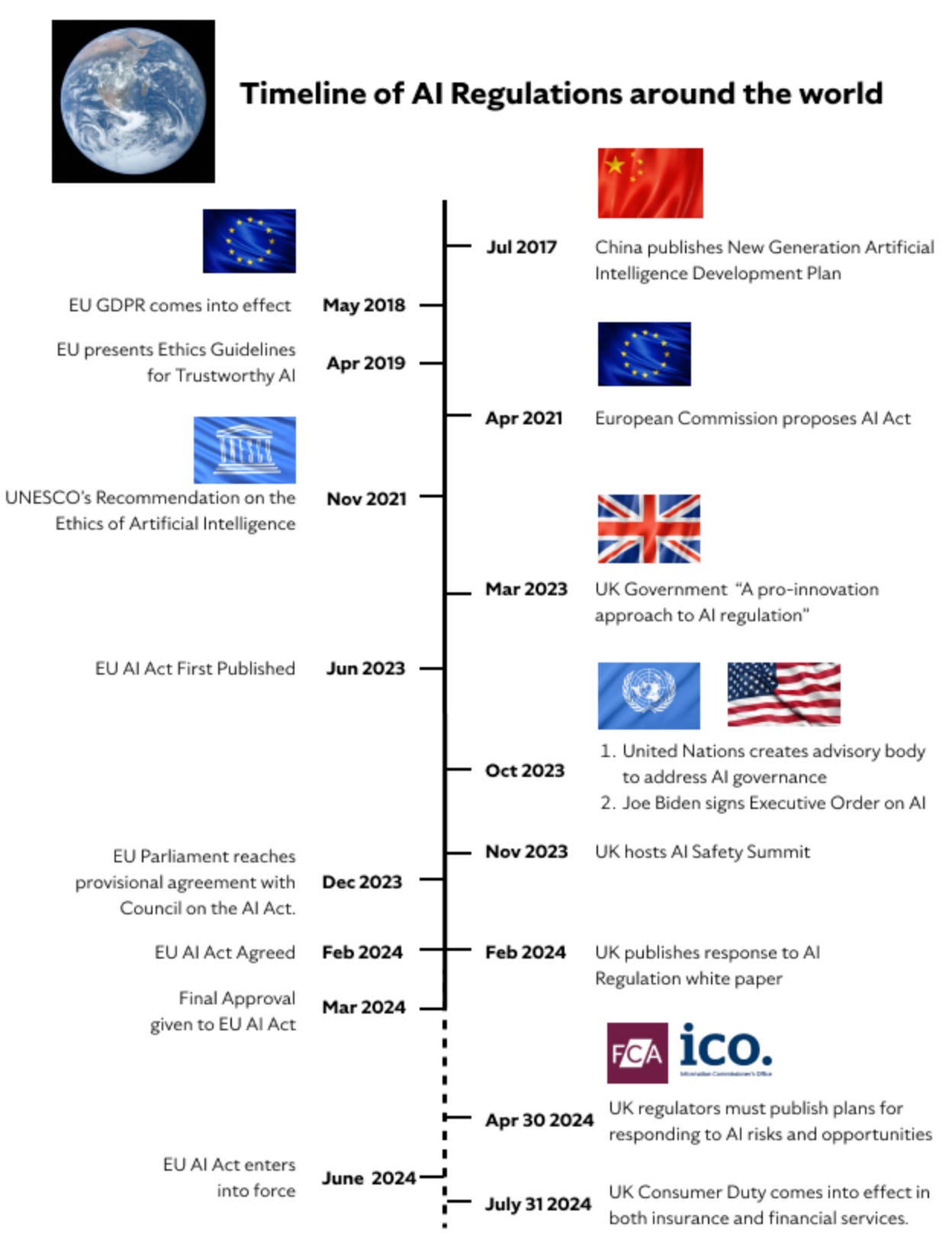
The efforts to develop policies around AI regulation is still in its early stages, and how that global AI governance will look remains an open question. While the US leads AI model development, a significant legislative action was only taken last October with the Biden administration signing an executive order on AI. In fact, China was the first country to issue enforceable interim regulations governing applications of AI. Meanwhile, with the approval of the European Union AI act, the EU has taken the lead in establishing a comprehensive regulatory framework for AI, which aims to regulate AI by categorizing potential risk and applying the appropriate regulations. It was first proposed in April 2021 and approved in March 2024. These are all remarkable feats in their own rights but all the major AI policy efforts are driven by developed nations, and developing nations, especially those in the global south, are and will be behind as they don't have the necessary resources, expertise, and structure to develop localized AI regulations. This disparity in AI governance capabilities could widen the digital divide and hinder the equitable distribution of benefits of AI.
AI policy guidelines must be adaptable
“the dominant policy prescriptions to encourage explainable AI are often rooted in Western perspectives.
- A Global South Perspective on Explainable AI by Jake Okechukwu Effoduh
Personally, I am in favor of localized AI policy but not all nations and regions can afford to work an AI policy from scratch so we need international frameworks that establish core principles, while allowing for adaptation to address specific needs of each country. As with most of AI, even the policies and guidelines with respect to AI safety, fairness and explainability are based on the narratives from the global north. Hence, the international frameworks must be a multi-stakeholder effort involving leaders, researchers, and representatives from diverse nations and communities around the globe. These frameworks should be grounded in research and an international understanding of what constitutes human well-being, fundamental rights, and equitable treatment across all races, genders, ages, abilities, and socioeconomic backgrounds.
Establishing universal standards and regulations for ethical AI governance is challenging given the disparities in technological access, cultural nuances, and economic conditions around the globe. However, it is important that we have international standards to make sure that AI systems are deployed responsibly even in nations that are not wealthy enough to devise their own policies and regulations surrounding AI.
Ethical alignment and benchmarking of models should begin early
With any innovation, the laws and policies governing them tend to follow only after a few concerning incidents have occurred and highlighted the need for regulation. As we develop AI systems at a speed and scale never seen before, we need a more proactive approach to tackling concerns in AI. Ensuring AI systems align with global values and goals requires collaboration from diverse stakeholders and careful ethical considerations, including (but not limited to) protecting users' privacy, mitigating biases, and ensuring safety and transparency throughout the lifecycle of the AI systems. Along with a clear alignment goal, proactive distilling of data, algorithms and and active red-teaming with experts from diverse domains and backgrounds must be in place to ensure that AI is adhering to the global ethical alignment.
“development of mature AI safety benchmarks that are both effective and trusted is not possible without the involvement of the community.
- Supporting benchmarks for AI safety with MLCommons, by Google
To fully leverage the benefits of AI systems we need effective and comprehensive AI safety benchmarks to rigorously test these systems before it is released for general access. The existing benchmarks (Eg. HELM, Big-Bench) focus on smaller subsets of issues with respect to fairness and safety, mostly limited to English and are not comprehensive. Development of comprehensive benchmarks that are inclusive require active and collaborative efforts from researchers, experts, developers and communities around the globe. As AI systems evolve rapidly in a "build-fast-break-fast" cycle, continuous testing and multiple iterations of the model is essential.
Conclusion
AI models not only exhibit human-like biases, studies have shown that they in fact amplify the biases that exist in our society. The data used to train these models is representative of the majority viewpoint and added with the implicit/explicit bias from the developers in selecting the data and processes to train the models, they continue to over-represent the dominant narratives. The proprietary nature of AI models makes understanding the sources of biases impossible and often leaves most of the world behind, especially, the already marginalized communities. In addition, the huge cost of training these models means the ability to train large AI systems is concentrated among a few players, making it challenging to ethically align these models to values and goals of the larger population. A collaboration from diverse stakeholders from around the globe is important in setting clear alignment goals before the development of AI. This collective approach helps ensure that AI is aligned for the people and their well-being, and it is not limited to profits and earnings for the corporations developing it.
In addition, the existing AI regulations are mainly developed from the perspective of the global north and the developing nations, especially those in the global south don’t have the resources or expertise to invest in research and development of AI policies. We need international AI regulation frameworks that can be adapted to the needs of developing nations with little effort. The responsibility of making AI responsible lies in all of us: industry leaders, researchers, experts, policy makers and the users of the system. A collective effort is needed to develop AI safety benchmarks and hold developers of these models accountable. In addition, establishing universal standards for ethical AI that can be adapted to the local community to ensure transparent and safe development of these models is critical.
Acknowledgements
Gwendolyn Gillingham Thank you for your feedback and help with editing this blog.
Rishabh Bhardwaj and Kshitiz Karki Thank you for pointing me to the reading materials.